
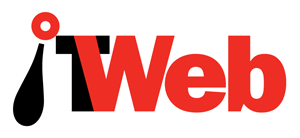
As analysis becomes increasingly crucial for business, data analysts, developers and business users alike are encountering stumbling blocks and disconnects in their quest for real-time, trusted data that delivers insights and action at the speed of business.
The answer to this challenge is automation across the data analytics pipeline.
The changes now taking place in the world of data analytics echo the evolution of DevOps. From a concept that developed in the 2000s, various toolsets evolved to make DevOps a reality.
Seen from the outside, DevOps delivered more continuous and consistent delivery of service. On the inside, DevOps technologies and toolsets were bringing automation to previously manual processes. To become truly transformational, hard lessons had to be learned and change had to take place in the realms of people and processes too.
This paved the way for the emergence of DataOps. First surfaced around five years ago, DataOps uses the same principles, seeking to make data teams more efficient and deliver better service to data consumers.
The ultimate goal of DataOps is to acquire greater business value from big data. It focuses on IT operations and software development teams and only works if line-of-business stakeholders work with data engineers, data scientists and data analysts.
Together, these data experts determine how best to get positive business outcomes from their data, while the line-of-business team members can point to what the company requires.
Underneath the DataOps umbrella are a number of IT disciplines − data development, data transformation and extraction, data quality, data governance and access control, for example.
The ultimate goal of DataOps is to acquire greater business value from big data.
However, the data analytics pipeline is complex and ever-changing. Automation in one siloed area cannot improve the overall data pipeline. The challenge industry faces is tying together multiple components, starting with understanding what data you have and building dashboards, through to analytics teams and business users.
DataOps in isolation tends to deliver data, but it doesn't necessarily enable people to use that data.
What organisations need to future-proof their analytics environment is active intelligence, which automates the whole pipeline from acquisition through to insight. It creates mechanisms that generate obvious visualisations. Active intelligence is based on continuous intelligence from real-time, up-to-date information with dynamic business content and logic.
The next evolution of this environment will be the incorporation of auto machine learning to give business users the ability to get even smarter insights, as well as progress towards automating the actions that are taken as a result of these insights.
Democratising data
As we discovered with the evolution of DevOps, automation cannot be successfully integrated without changing people and processes.
Key areas where people and processes could slow adoption and undermine the benefits of automation are resistance to change, and a fear that automation is a threat. The old-school mindset of IT and data teams owning the data will have to change as business users quickly become more data literate.
With automation across the data analytics lifecycle, data is not only democratised, it is also trusted and available in real-time to all the business users who need it. Instead of this being a threat to the traditional data stewards, it makes work simpler.
At the point where data is acquired, we don't want humans to have to sit worrying about new data fields or obsolete data fields − the system should simply manage it for them.
Where we deliver data out of systems, we want to pull through obvious metadata in an automated way, organise it and get it into a usable catalogue, so that people no longer have to document every last step.
They should be allowed to add and extend metadata, making it easy to find from two sides. The DataOps and analytics teams need to be able to track the onward journey of the data from the catalogue, and everyone should be able to trust the data lineage.
Moving to a state of active intelligence is crucial if business strategy is to be linked with data strategy.
Business users need to know their data is accurate, updated and trusted for analysis. They need powerful tools to find, understand and use data based on their unique needs and security credentials.
Data engineers need to quickly add new data sources and ensure success across the entire pipeline − from real-time data ingest to refinement, provisioning and governance. At the same time, data protection features must be easy to administer, even in very large settings with many users, data sources, or complex infrastructure.
The move to automation is a natural progression to address these needs. We need to start educating everyone involved in analytics − from analysts and developers of dashboards through to business people − so they understand that to fully benefit from active intelligence, they need to make automation the backbone of their data analytics pipeline.
In my next article, I will explain why application automation is necessary to drive dynamic actions.